🎓 Building a Self-Improving Customer Success Agent
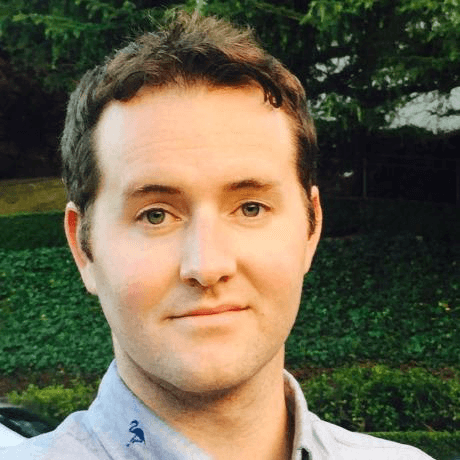
Chris Jones
on Apr 3, 2025
Editor's note: this post is intended for the audience of TypeScript developers.
Technical content rating: 🎓 basic coding literacy assumed
In the universe of AI bots, you could say that a Customer Success (CS) Agent is the equivalent of a "Hello world" program: it answers questions from a knowledge base. Why should you care about this one?
Our goals were to:
Enable you use our CS Agent as a "reference app": a starting point to remix and customize in order to build your own, more interesting agent
Show off our platform features that we're excited for you to use to build your own apps; see below
Let you deploy CS Agent in order to kick our tires as a platform, and hopefully get some value out of, perhaps by integrating with internal MCP servers or other apps
What does the CS Agent reference app do?
You have a Customer Success (CS) Team, and you have an Eng Team. They probably share a Slack channel where they've had previous discussions about support requests, and shared relevant links and documents.
When you invite the @CSAgent bot into your channel, it will:
ingest all previous message threads to make them available for generating answers to future questions
ingest documents and scrape links as additional context
start responding to questions that @-mention it
So CS Agent becomes your frontline escalation handler, before you need to loop in humans:

Note that in its reply to the support question, the CS Agent links to the original Slack threads and documents that it generated the answer from. Hallucinated support responses are not helpful.
And as shown in the bottom-right of this screenshot, humans can react 👍 and 👎 in response to the bot's answer. This allows it self-improve over time by replying more like 👍 responses, and avoiding 👎 responses. We'll have more to say about self-improvement in future posts.
And, your CS team members can keep posting messages back and forth with the CS Agent for as long as it takes to craft a great reply to the support ticket — as opposed to a minimal reply to be polite about demands on time from humans.
How is the the slackbot built?
Our CS Agent takes the shape of:
A DeepStructure application running on our managed DeepStructure Cloud
At the core, an AI Assistant named
cs-agent
that draws onvector_store
s to provide relevant context to answersPrebuilt DeepStructure "slackbot" component that maps Slack updates into "reactive events"
TypeScript workflows that are triggered by the reactive events from Slack and do useful things in response
The code comprising the slackbot is available in our reference-apps
GitHub repository. You can deploy it, customize, remix it with other apps, whatever you want.
[Editor's note: as of 2025-04-02 we're still in limited preview mode, so if you would like access, please email us at hello@deepstructure.io!]
What does the cs-agent
AI Assistant do?
The cs-agent
Assistant and its vector_store
s are built on top of the OpenAI Assistants API. Assistants give you a simple way to coordinate models, prompts, message threads, file retrieval, and tool calls without having to know much about how it all works. And you don't need to run the code yourself — it's all behind a (relatively) simple API.
Here's the configuration of the cs-agent
, which may look familiar to you:
But here at DeepStructure, we actually built our own, clean-room implementation of the Assistants API using our core TypeScript SDK. There's no "magic sauce" in the implementation. Why would we do that?
DeepStructure Assistants give you a number of benefits:
All the internal operations of the Assistant, from top-level API calls down to the lowest-level details of running vector-store retrieval, are exposed to you via DeepStructure's observability system. You never have to wonder why an Assistant produced the result it did; you can simply trace all the dataflows yourself.
You have the optionality to work with any model provider or vector store. Our Assistants understand OpenRouter-style model names, so you can for example specify
model: "anthropic/claude-3.7-sonnet"
to experiment with a different state-of-the-art LLM for your use case.All the API calls and dataflows are processed within the context of your application, inside your cluster tenant. No data leaves your application's boundaries unless you allow it to. (And if you self-host the platform, no data need ever leave your VPC!)
We've extended the Assistants API in several ways we've found useful, including: (i) feedback on Assistant runs; (ii) lower-latency tool calls; (iii) fallback models for when you hit rate limits or schema validation errors
We've added higher-level wrappers on top of the raw Assistants API that make it more convenient to work with; we show a few of them below. Most useful of all, we model
vector_store
s likeBlobStore
s, so that you can work with them in a similar way as you would work with S3 or Google DriveBlobStore
s by getting, putting, and listing objects.
And because our implementation stays faithful to the OpenAI API, if you're already using Assistants, you can switch your existing client over to our implementation by changing a single line of code:
How does the Slack integration work?
Typically, you would think of integrating with Slack via their API and webhooks to notify handlers in your service. But these are low-level plumbing that are just a means to the end of what you're really trying to do:
react to events of interest like threads being created, your bot being mentioned, new messages in thread, emoji reactions to messages, files uploaded
process the old messages in the channel, like how you would list and get objects from a
BlobStore
Building on top of Slack's raw API and webhooks, you might spend so much time on the low-level plumbing that you may not get around to pondering:
what happens if my bot-mentioned event handler, for example, throws an exception while processing a message?
what happens if my service is down for a couple of hours? What happens if Slack is down for a couple of hours?
Our less-technical friends who build no-code automations don't have to worry about these kinds of degenerate cases. The no-code engine handles the details of executing their workflows reliably. Why do we developers have to worry about these things?
DeepStructure's approach is to let you have your cake and eat it too: build a v1 quickly without having to learn a new programming model; and end up with a robust system from the get-go.
As a concrete example, here's code that responds to a "bot mention" in Slack (e.g., someone types "@CSBot how can the user switch to dark mode?"):
We hope you read this code and think, "well that's just regular TypeScript with an EventEmitter and some Slack and Assistants helpers, why should I care?" Except there's that C()
wrapper function …
What DeepStructure has actually done here is pulled some sleight-of-hand on you, and the C()
thing is the "tell":
We have "upgraded" the
EventEmitter
pattern into aReactiveEventEmitter
patternInstead of dispatching events to regular TypeScript function handlers,
ReactiveEventEmitter
dispatches them to resilient TypeScript workflows that can run in parallel across different nodes, and automatically recover from transient failures(We've done some build and packaging gymnastics to make this mostly transparent to you, except for …)
… where you see the
C()
helper lifting a regular TypeScript function into aComponent
, a single step within a workflow that can be scheduled on an arbitrary node, and automatically recover from errors
And congratulations — just by writing this reactive-event-handler workflow, you've also built comprehensive observability for it too! You can trace all the raw data flows from the triggering of the "mention"
event, all the way through sending your reply to Slack, using DeepStructure's observability system. And this includes tracing into all the inner steps of the Assistant run.
In conclusion
We'll have more to say about these details in future posts. For now, we want to remind you that we've shown you the exact same set of primitives that we used to build our Assistants implementation. If you're building anything that looks like Assistants, we hope this will be a useful, and obvious, programming model for you too.
The mission of DeepStructure is to solve the hardest problems of building robust AI systems for you, so that can leverage your skills as a TypeScript developer to build the features your users want — leave the plumbing to us.
We hope you find the CS Agent reference app a useful place to start, and we always value your feedback! Please drop us a line at hello@deepstructure.io.
Don't miss these
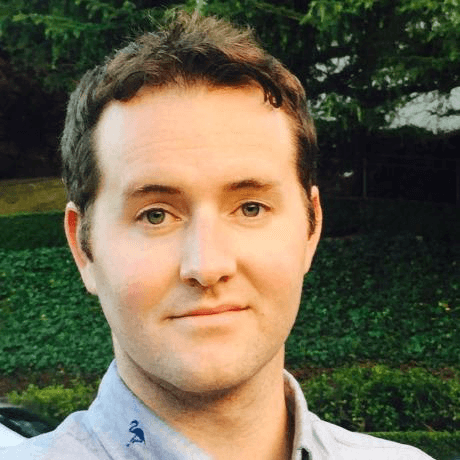
Chris Jones
🎓 Shortcut to SOC 2: Use AWS Identity Center for Auth
AWS Identity Center gives you role-based access control and SSO with low effort, giving you a leg up on the road to SOC 2 compliance

Andrew Gaul
🎓 Alerts Agent on the DeepStructure Platform
DeepStructure developed an application to address our internal IT oncall needs using our TypeScript-native AI platform. In this post I will show how we combined MCP, Agents, and Slack (MÁS stack) to solve a real-world problem. While this is a specific demonstration, the DeepStructure platform allows external developers to mix-and-match MCP servers, different models, and a variety of frontends to create their own agent workflows.
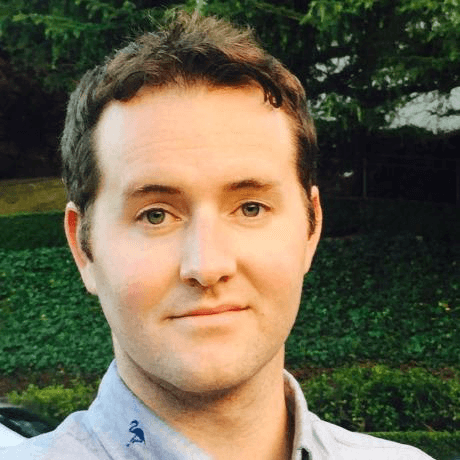
Chris Jones
🎓 Thinking about MCP as "npm for LLMs"
We describe three parallels between MCP and npm: (1) sure, you _could_ build the tools yourself, but now you don't have to; (2) the integrations become the vendor's problem, not yours; (3) even though the ecosystem isn't uniformly high quality, there's still value for developers who follow best practices